
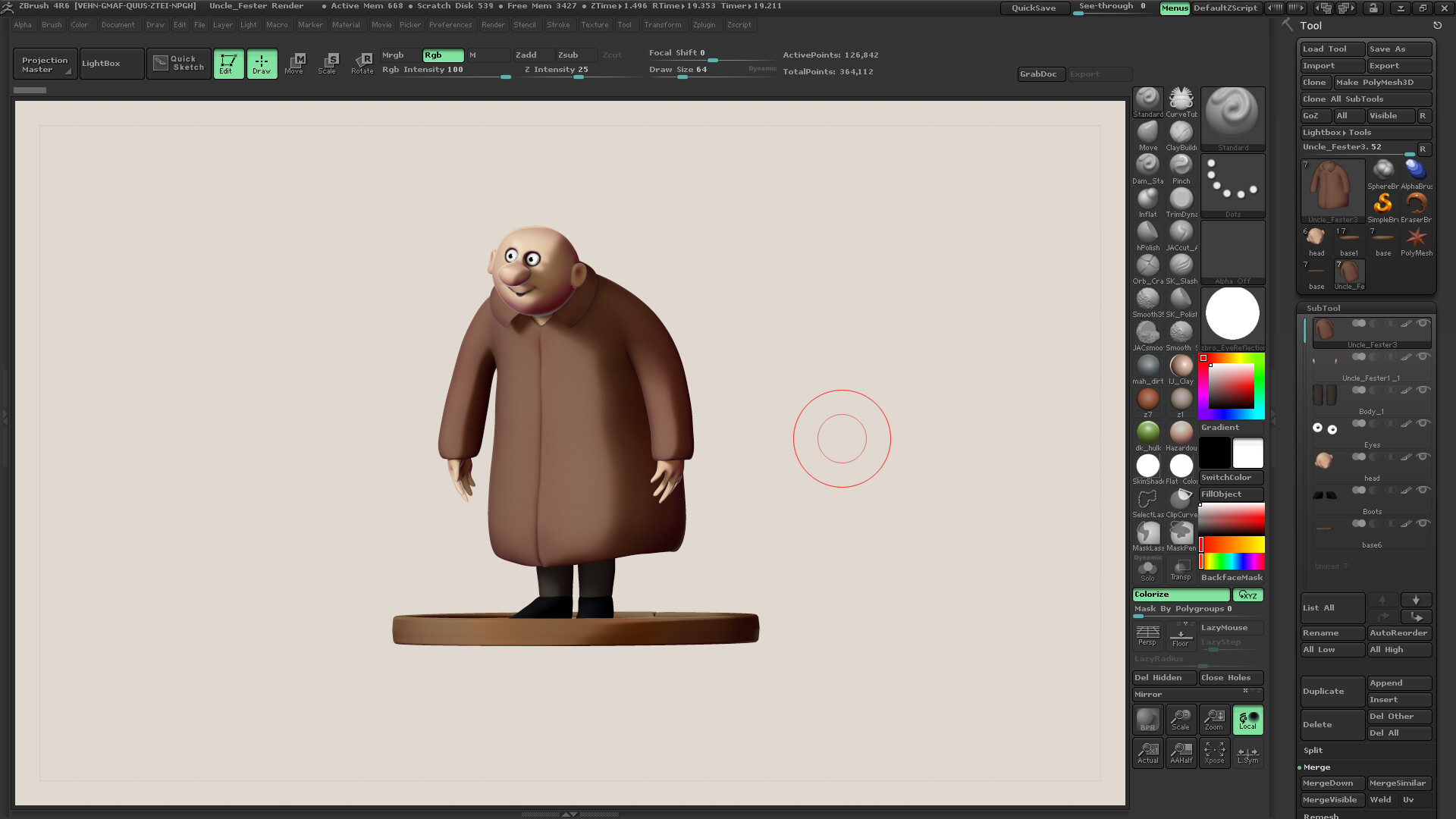
Root finding comes up in analytics where we answer enterprise questions. Regression is how the enterprise gets its situational awareness. It’s the bread-and-butter of data fusion. The regression problem is obviously crucial to data science.

By some miracle of Nature, or cosmic mathematical coincidence, there are three extremely important problems that end up being identical:įinding the best fit to a set of data (regression),įinding the places where the a system or function takes a certain value (root finding),įinding the minimum or maximum value of a system or function (optimization). But we promised, at the end, to illuminate the distinctions between optimization and control, starting with a more structured discussion of optimization. Control: Uses the values of enterprise variables and answers to enterprise questions to inform decisions and actions to further enterprise goals.įor that discussion, we treated control and optimization as the same thing and used the word "control" as shorthand for both.Analytics: Applies business rules to variables of interest giving answers to enterprise questions.Data Fusion: Transforms observations of the world into estimates of variables of enterprise interest.
#Watch demon slayer movie mugen train series
So part 1 of this series provided a taxonomy of data science that aligns well with the Data/Information/Knowledge/Wisdom Pyramid, dividing data science into three complementary components: Part 4 (pending): Deals with difficult issues in control in the real world things like selecting the best controls in the absence of important information, or in the presence of deceptions in the operating environment.Part 3: Moves into the theory of control.regression analysis) as the same problem. Identifies optimization and data fitting (e.g. Part 2 (this part): Deals with a taxonomy of optimization.
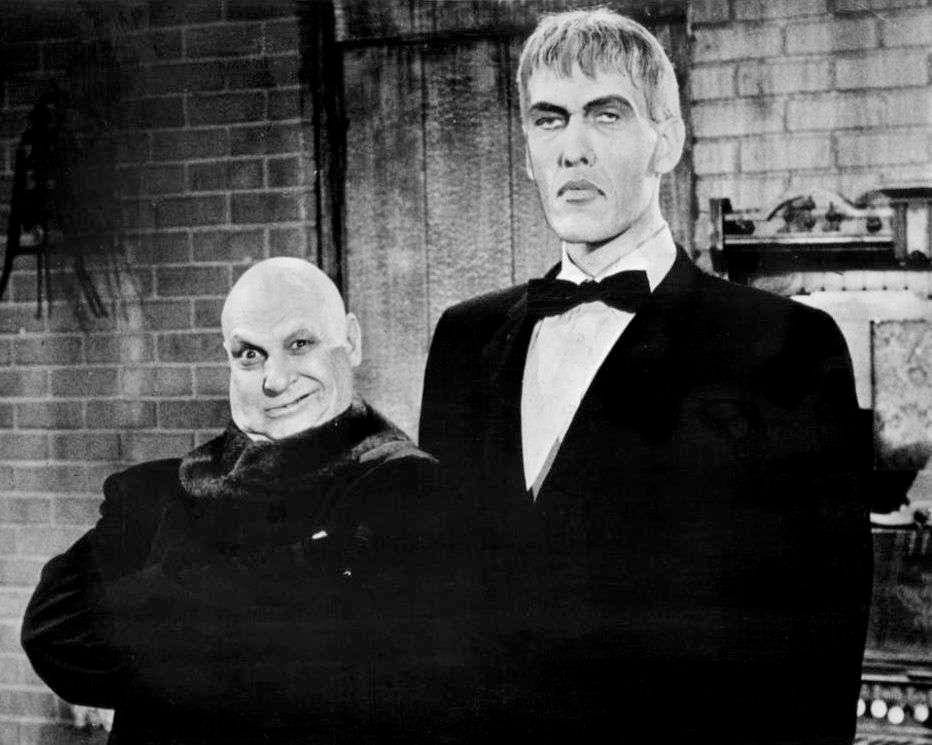
It also identifies control as a key component of both data science and simulation science.
